
A bank has to make a regulatory change every 12 minutes. That’s some task. A bigger question must also be: how can a regulatory check that a bank is compliant with their regulatory changes, if their regulations change every 12 minutes are run to 1,000’s of pages?
The answer is they can’t, but they don’t have to. They only need to check that some of the things that count are being complied with, such as data reporting on time and spot checks to see that the compliance people are in position doing what they’re charged with doing: ensuring compliance.
There’s then a lot of trust between the regulator and the bank that they are doing everything with the right intent and action. That’s why, preceding the global financial crisis, many regulatory checks were being made only once a year or not at all. A clear example of this failure was Northern Rock*:
Insofar as the FSA undertook greater “regulatory engagement” with Northern Rock, this failed to tackle the fundamental weakness in its funding model and did nothing to prevent the problems that came to the fore from August 2007 onwards. We regard this as a substantial failure of regulation.
But there are many others. After all, this complex minefield of regulatory compliance is just as hard on both sides of the fence. Some think this is being solved with technology. For example, the Austrian government has made a first move to have real-time regulatory access to their banks’ systems. That could be a game changer if the regulator knows what they’re looking for from that real-time regulatory access. And this is where the meeting on Governance, Risk and Compliance (GRC) got interesting, as the discussion was mainly about using machine learning and artificial intelligence to mine bank data for compliance. This is a process that includes both the regulated and the regulator developing a joint language and standards for regulatory compliance, then applying systems and technologies to monitor, mine and measure compliance, reporting any issues and alerts.
Sound impossible?
Not really. The language and standards for example, are being actively worked upon in Ireland by the Governance, Risk and Compliance Technology Centre (GRCTC). The GRCTC has started with a Financial Industry Regulatory Ontology (FIRO). FIRO is a family of ontologies that enable efficient access to the wide and complex spectrum of regulations through formal semantics. OK, so we’re already disappearing into academic over-the-head terminologies, so let’s just elaborate a little on two of the terms mentioned here: ontology and semantic.
An ontology is a set of concepts and categories in a subject area or domain that shows their properties and the relations between them. This is the start point for building a semantic regulatory system.
Wikipedia defines semantic as the linguistic and philosophical study of meaning in language, and also in programming languages, formal logics and semiotics. It focuses on the relationship between signifiers like words, phrases, signs, and symbols; and what they stand for.
Putting together the ontology of regulatory monitoring and a semantic intelligence, and you can rock and roll. What is the semantic system of technologies involved here? Well, all of my favourites:
These are the building blocks of the semantic web, something that has been dreamed of for over a decade and now, possibly, is coming to fruition. The semantic web is being built by the World Wide Web (W3) Consortium, the main international standards organisation for the web. They define the development of the semantic web as follows:
In addition to the classic “Web of documents” W3C is helping to build a technology stack to support a “Web of data,” the sort of data you find in databases. The ultimate goal of the Web of data is to enable computers to do more useful work and to develop systems that can support trusted interactions over the network. The term “Semantic Web” refers to W3C’s vision of the Web of linked data. Semantic Web technologies enable people to create data stores on the Web, build vocabularies, and write rules for handling data. Linked data are empowered by technologies such as RDF, SPARQL, OWL, and SKOS.
Oooh, this is doing my head in.
Yes, it’s complex because we have an ontology of regulatory nomenclature, that will be semantically linked through a combination of mixed technologies from AI to blockchain, to enable machines to learn how to monitor regulatory compliance, rather than humans.
Intriguingly, in this discussion, I’ve not heard one person who believes there will be no humans in this process, as you need human logic and insight to see regulatory bubbles forming. Catching those bubbles before they explode is the regulators role. Anyways, going back to the GRCTC, what are they really trying to achieve?
The financial services regulatory domain is being modelled in FIRO through an innovative approach that includes the semantic analysis of legal texts and rules by legal and financial subject matter experts (SMEs), their translation into a structured natural language, and the mapping of this regulatory natural language and rules this into machine language by ontology engineers. Regulatory ontology development is a non-trivial and challenging task … thus, a regulatory ontology such as FIRO can help:
Financial services companies to monitor, assess, and apply a multitude of regulations within and across regulatory domains to business processes and data;
- Model the regulations to help simplify their consumption;
- Make it simpler for enterprises to map GRC policies onto regulations and perform Regulatory Change Management;
- Help organisations keep abreast of the ramifications of complex interacting regulatory rules and policies;
- Reason over regulations to identify risks and compliance issues;
- Contribute to the emergence of SMART Regulation.
There’s quite a lot more on this area if interested, as it’s all about the rise of RegTech. What I’ve just described – the semantic regulator – is obviously one big ticket item of development, but there are six more. These were detailed in a recent Institute of International Finance (IIF) report on RegTech.
Here’s their summary of the RegTech use case areas and technologies:
- Risk data aggregation as required for capital and liquidity reporting, for RRP and for stress testing, implies the gathering and aggregation of high quality structured data from across the financial group. It is complicated by definitional issues and the use of incompatible and outdated IT systems.
- Modeling, scenario analysis and forecasting as required for stress testing and risk management is increasingly complex and demanding in terms of computing power and labour and intellectual capacity, due to the vast array of risks, scenarios, variables and methodological diversity that needs to be included.
- A bottleneck in monitoring payments transactions (particularly in real-time) is the low quality and great incompatibility of transaction metadata churned out by payments systems. This complicates automated interpretation of transactions metadata to recognize money laundering and terrorism financing.
- Identification of clients and legal persons, as required by know-your-customer regulations, could become more efficient through the use of automated identification solutions such as fingerprint and iris scanning, blockchain identity, etc.
- Monitoring a financial institution’s internal culture and behaviour, and complying with customer protection processes, typically requires the analysis of qualitative information conveying the behaviour of individuals, such as e-mails and spoken word. Automated interpretation of these sources would enable enormous leaps in efficiency, capacity, and speed of compliance.
- Trading in financial markets requires participants to conduct a range of regulatory tasks such as margins calculation, choice of trading venue, choice of central counterparty, and assessing the impact of a transaction on their institution’s exposures. Automating these tasks will ensure compliance and increase the speed and efficiency of trading.
- Identifying new regulations applying to a financial institution, interpreting their implications and allocating the different compliance obligations to the responsible units across the organization is currently a labour-intensive and complex process, which could be enhanced through automated interpretation of regulations.
The report identifies several recent technological and scientific innovations and describes how they are, or could be, applied as RegTech to help financial institutions comply with financial regulations.
- Machine learning, robotics, artificial intelligence and other improvements in automated analysis and computer thinking create enormous possibilities when applied to compliance. Data mining algorithms based on machine learning can organize and analyse large sets of data, even if this data is unstructured and of a low quality, such as sets of e-mails, pdfs and spoken word. It can also improve the interpretation of low-quality data outputs from payments systems. Machine learning can create self-improving and more accurate methods for data analysis, modelling and forecasting as needed for stress testing. In the future, artificial intelligence could even be applied in software automatically interpreting new regulations.
- Improvements in cryptography lead to a more secure, faster and more efficient and effective data sharing within financial institutions, most notably for more efficient risk data aggregation processes. Data sharing with other financial institutions, clients and supervisors could equally benefit.
- Biometrics is already allowing for large efficiency and security improvements by automating client identification, which is required by know-your-customer (KYC) regulations.
- Blockchain and other distributed ledgers could in the future allow for the development of more efficient trading platforms, payments systems, and information sharing mechanisms in and between financial institutions. When paired with biometrics, digital identity could enable timely, cost efficient and reliable KYC checks.
- Application programming interfaces (APIs) and other systems allowing for interoperability make sure that different software programs can communicate with each other. APIs could, for example, allow for automated reporting of data to regulators.
- Shared utility functions and cloud applications could allow financial institutions to pool some of their compliance functions on a single platform, allowing for efficiency gains.
* House of Commons Treasury Committee: The Run on the Rock
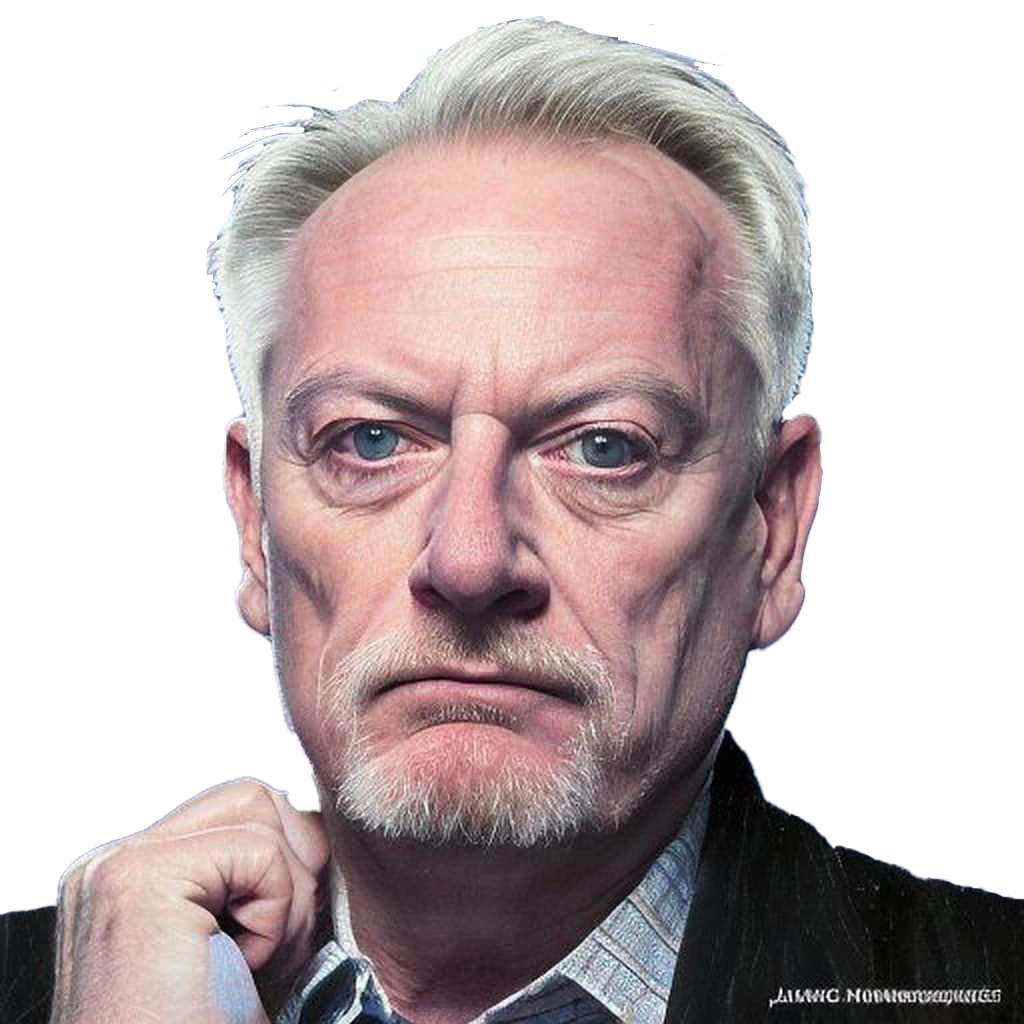
Chris M Skinner
Chris Skinner is best known as an independent commentator on the financial markets through his blog, TheFinanser.com, as author of the bestselling book Digital Bank, and Chair of the European networking forum the Financial Services Club. He has been voted one of the most influential people in banking by The Financial Brand (as well as one of the best blogs), a FinTech Titan (Next Bank), one of the Fintech Leaders you need to follow (City AM, Deluxe and Jax Finance), as well as one of the Top 40 most influential people in financial technology by the Wall Street Journal's Financial News. To learn more click here...